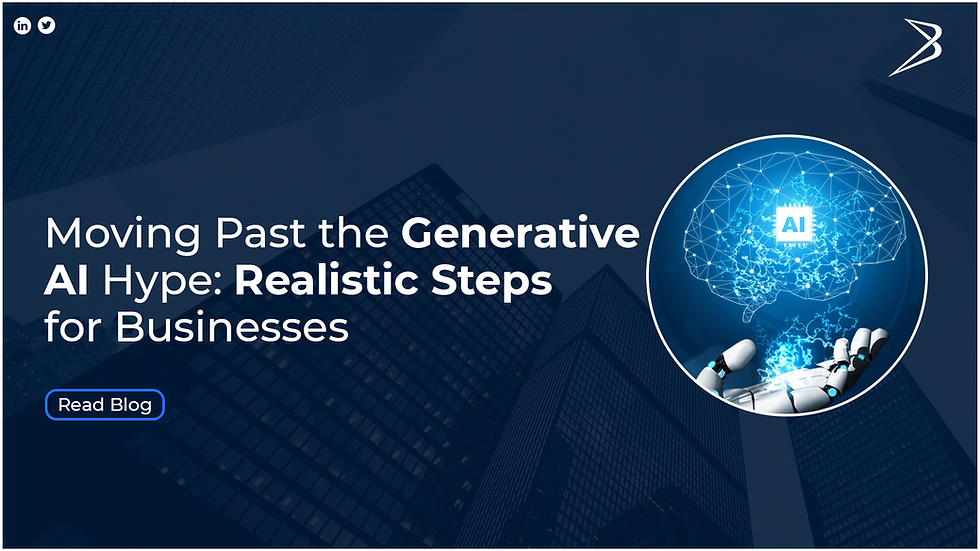
Introduction
It seems that the hype surrounding Generative AI has started to cool, settling into a more realistic phase.
This feels like a good time to share some reflections from our experiences at Beyond, working with an exceptional range of clients across Retail, Travel, Healthcare, and Financial Services.
Generative AI, with its remarkable ability to create everything from text and images to code, is among the most hyped technologies of our time.
I remember the gradual excitement as the internet gained traction. In contrast, Generative AI—though part of the broader, longstanding field of Artificial Intelligence—appeared to reach peak interest almost overnight.
Now that the initial enthusiasm has waned, businesses are stepping back to focus on practical strategies for developing and integrating this technology into their business models and systems.
McKinsey estimates that generative AI could add between $2.6 trillion and $4.4 trillion annually to the global economy. Yet, only 11% of companies report success in scaling it beyond pilot stages.
At Beyond, this statistic comes as no surprise. Nearly all our clients across sectors like retail, travel, and healthcare encounter operational challenges and integration obstacles when aiming to scale ambitious AI initiatives.
Here are some reflections from Team Beyond on how executives can pragmatically navigate this evolving landscape.
Understanding Generative AI’s Potential and Limits: Expanding Beyond the Initial Excitement
The generative AI journey often begins with experimentation. Typically, a forward-thinking business unit—often in marketing or the data team—proposes ideas to enhance customer experience or drive back-office efficiencies.
At Beyond, we’ve worked with clients across diverse sectors to pilot initiatives in areas like automated product recommendations in retail and travel, and automated data insights in healthcare.
However, we have also observed that achieving meaningful business impact from these pilots requires a clear-eyed view of AI’s potential, its limitations, and a dose of pragmatism.
Generative AI excels in specific areas—such as boosting productivity in customer support or creating marketing content at scale—but not every business problem is suited to this technology, and it’s easy to overestimate its capabilities.
In retail, for example, AI-driven product recommendations can enhance customer engagement, but only if supported by strong data architecture and customer insights.
For healthcare, strict governance around patient and staff privacy and data security must be considered before generative AI can be safely applied across the value chain.
From our experience, the key is identifying where generative AI genuinely adds value and focusing efforts accordingly, rather than spreading resources thinly across unproven use cases.
Critical Actions for Technology Leaders: Guiding AI Adoption Across the Enterprise
For technology leaders, generative AI adoption goes beyond simply implementing a new tool; it’s closer to orchestrating a transformation.
Based on our experiences at Beyond, we recommend several key actions for CIOs and CTOs:
Define Your AI Strategy and Risk Boundaries: Set a clear AI strategy with well-defined risk parameters, ensuring alignment across the business, especially in sensitive areas like customer data privacy.
Collaborate on New Business Models: Work with senior leaders to explore AI-driven opportunities that could reshape business models, such as hyper-personalisation in customer journeys or new revenue streams.
Upgrade Technology Foundations: Modernise IT infrastructure and data pipelines to support scalable AI deployment, integrating AI seamlessly with existing systems.
Upskill Your Team: Invest in targeted training across roles to ensure employees understand and can leverage AI tools effectively.
Embed AI Governance and Ethical Safeguards: Implement governance frameworks to manage AI risks, from privacy to potential biases, ensuring compliance and ethical use.
Building Robust Architecture and Data Pipelines: Foundations for Scalability
A robust technical and data foundation is essential for generative AI to deliver value.
These models require vast amounts of quality data, making it necessary to upgrade data management systems to handle both structured and unstructured data.
Beyond has assisted companies across sectors, enabling them to evolve their data architectures for smooth AI integration.
For example, in retail, models might need real-time access to inventory levels, customer preferences, and purchasing trends to generate personalised product recommendations.
Without this foundational infrastructure, AI outputs risk being irrelevant or, worse, inaccurate.
Essential Components:
Context Management & Caching: Provides AI models with relevant data, expediting responses.
Policy Management: Controls data access, crucial in regulated industries like healthcare.
Model Hub & Prompt Library: Centralises approved models and prompt templates, enabling consistent AI usage across departments.
MLOps Platform: Facilitates efficient model deployment, monitoring, and adjustments as business needs evolve.
Skills and Culture Shift: Upskilling the Workforce
Generative AI’s potential reaches far beyond the IT team, impacting nearly every department. At Beyond, we view AI adoption as a cultural shift rather than solely a tech initiative.
While some hype has focused on AI replacing jobs, we see it differently: generative AI can serve as a productivity tool, enhancing current roles or efficiently handling repetitive tasks.
For example, retail employees can be trained to interpret AI-driven product recommendations, enhancing customer interactions.
In healthcare, administrative staff can use AI to streamline data entry, freeing up time for patient care.
By fostering a culture of learning and experimentation, businesses can ensure AI is embraced across the organisation, leading to higher productivity and smoother adoption.
Embracing Responsible AI and Risk Management: Mitigating AI-Specific Risks
With the power of generative AI comes a responsibility that must be taken seriously.
At Beyond, we have observed that as companies explore AI-driven insights, personalisation, and automation, they must also address risks like bias, privacy, and security.
AI’s inherent risks—including "hallucinations" (where models generate incorrect or random responses) and data privacy issues—demand rigorous safeguards.
For example, in healthcare, stringent data privacy regulations require tight controls on patient and staff data access.
In retail, ensuring fair treatment in AI recommendations for credit services necessitates careful bias mitigation.
By establishing ethical guidelines and regulatory safeguards, companies can maintain trust with both staff and customers while minimising liabilities.
Controlling Costs: Managing Development vs. Operational Expenses
Generative AI can be costly to develop and operate, especially given the need for constant updates and monitoring.
At Beyond, we work with clients to develop cost management strategies that prioritise high-impact AI use cases while containing operational expenses.
Understanding the cost structure of generative AI is essential for keeping projects within budget and maintaining support from financial leadership.
Conclusion: Moving Forward with Generative AI
As generative AI moves beyond its "honeymoon phase," companies should focus on sustainable, value-driven applications.
At Beyond, we’ve seen how generative AI can transform businesses when approached strategically, from improving customer engagement in retail to enabling personalised experiences in healthcare.
This journey requires disciplined focus on high-value use cases, careful cost management, and a proactive approach to risk and compliance.
If you would like our help with your Generative AI plans, feel free to reach out for a conversation at info@puttingdatatowork.com.