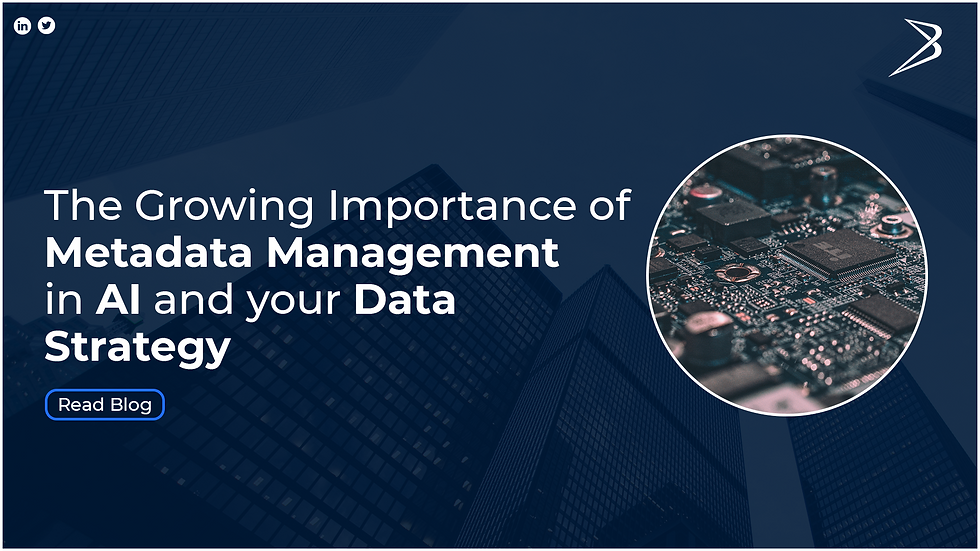
Introducing Metadata management and its role in data and AI
Working in our world of data, normally when you mention Metadata Management (MDM) you expect your audience’s eyes to glaze over and for them to suddenly start finding more interesting things to focus on, such as their phone.
But recently that has been changing and it’s them probing us for more insights and advice on what they need to be doing to sort out their data quality and fix their MDM.
Why the sudden interest? It’s all down to the rapid interest in all things to do with Artificial Intelligence (AI) and more specifically ChatGPT.
Now that everybody in the business is suddenly fixated on using AI they are fast discovering that everything they’ve been told for years about why they need to take data quality seriously - briefly summarised as “Sh*t in - Sh*t Out” is now more important than ever.
In the rapidly evolving world of AI, having an effective set of metadata management processes, and tools to support these, has become a crucial capability for businesses that want to harness the full potential or promise of AI.
AI, and in particular generative AI which relies on doing a lot more processing and learning by itself without humans getting so involved in the data inputs and logic per se, need good data.
Not just so they can function effectively but now, more than ever, so they aren’t spitting out rubbish because they have been learning on flawed data. As AI systems, particularly generative AI (GenAI) and large language models (LLMs), become a bigger part of day to day business processes, the need for a robust approach to metadata management cannot be ignored.
Despite its critical importance, which has always been there, metadata management has often been overlooked. It’s suffered from the being at the less sexy end of data, not just because of its name (its hardly inspiring), but also due to its complexity and the substantial effort required - both from a technology perspective, but mainly because it relies largely on human behaviour and process change to be effective.
As AI grows and grows, managing metadata effectively is becoming increasingly vital.
What's the difference between Metadata Management and Masterdata Management?
To the uninitiated the two sound a bit too similar and often cause confusion. A simple explanation is as follows:
Metadata Management: Think of metadata management as the detailed labeling system for all your data. It provides critical context—like where the data came from and how it's been used—making it easier to find, understand, and govern your data effectively. This ensures transparency and compliance across all data operations.
Master Data Management (MDM): MDM is about creating a single, accurate view of your key business entities such as customers, products, and suppliers. It consolidates data from multiple sources to ensure consistency and quality, which enhances decision-making and operational efficiency.
In Brief:
Metadata Management helps you understand and govern your data.
MDM ensures you have a consistent, reliable view of your core business data.
Both are essential for leveraging your data effectively and making informed business decisions.
Understanding Metadata and Its Role in AI
Metadata is often described as the “data about data”. It quite literally is the data that explains the data so that data engineers, systems integrators, data scientists et al. know what the data they are using represents and therefore how to use it.
In essence it is providing the essential context for understanding and using data effectively including in it information such as the source of the data, its structure, creation date, and usage history.
In Data Analytics, Data Science, AI and machine learning, metadata is pivotal throughout the process, from identifying what data is available to consider as part of a solutions or model but also critical for training models themselves and ensuring data quality and integrity.
With AI, and Generative AI in particular, generating ever more vast amounts of data, managing the metadata that sits behind it becomes essential for several reasons:
Improving Data Quality and Consistency: Metadata helps maintain data quality by providing a comprehensive audit trail and ensuring data consistency across different systems and applications.
Facilitating Data Integration and Interoperability: Metadata enables seamless data integration and interoperability, allowing diverse data sources to work together efficiently.
Enhancing Data Discoverability and Usability: Metadata makes data more discoverable and usable by providing detailed descriptions and context, which are essential for data analysts and scientists ensuing they are making the best use of data available.
Leveraging AI Expertise: Unless you happen to to work for a very large, extremely wealthy organisation that can attract and retain the best Data and AI talent, then you are going to be looking for outside help. Without a strong foundation in your MDM the job for external consultants or data scientists becomes much bigger, much harder, much more expensive and riskier than it really needs to.
The Challenges of Metadata Management
Despite its importance, metadata management is often seen as a daunting task. The challenges include:
Volume and Complexity: The sheer volume of metadata generated by modern data systems can be overwhelming and historically this volume has been considered a bit of a distraction to the point that it has often been ignored and left to effectively ‘get lost’ in the general technology eco-system. Managing this data effectively requires a level of sophistication in terms of tools and processes that IT or Data teams have traditionally given a bit of wide berth - often with a bit of a “what they don’t know, won’t hurt them attitude” with regards telling the wider business.
Lack of Standardisation: Metadata standards vary widely across industries and organisations, making it challenging to implement a uniform metadata management strategy. This is really obvious when your business has grown through acquisitions and mergers and where legacy systems and processes have been smashed together and endless work arounds have been created by the business.
Resource Intensiveness: Effective metadata management requires significant resources, including skilled and experience business personnel as well advanced technologies. Most often in our experience MDM is an after thought and requires a huge amount of forensic investigation and validation that also requires a lot of time and effort from business users who can be the only people who really understand the context of the data in question.
Leveraging Active Metadata for AI
Active metadata is metadata that is continuously updated and leveraged in real-time, is crucial for increasing the potential of AI systems.
Examples of Active Metadata
Real-Time Data Quality Checks | Active metadata can automatically check and report on data quality in real-time. For example, it can monitor and flag incomplete entries in a customer database, alerting the data team to missing phone numbers or email addresses. |
Data Usage Tracking | Active metadata logs who accessed which data and when. For instance, it can show that a marketing manager accessed sales data on Monday morning, providing transparency and security insights. |
Automated Data Classification | Active metadata tags data based on its sensitivity. For example, it can label customer payment information as "sensitive" to ensure it’s handled securely and complies with privacy regulations. |
Performance Monitoring of AI Models | Active metadata tracks how well AI models are performing. For example, it can report that a recommendation algorithm's accuracy has dropped, prompting a review and adjustment. |
Contextual Information | Active metadata adds context to data. For example, it might note that a sales spike occurred during a promotional event, helping analysts understand trends better. |
By using active metadata, assuming you are managing it effectively so it is usable, businesses can create dynamic data environments that further improve the capabilities of their AI and the management/operations of it. Three examples below are highly relevant today:
Enhancing AI Model Training - Active metadata enriches AI models with important context which helps them learn better. This is a bit like giving a student not just a textbook, but also the notes and highlights that explain key concepts, making the outcomes smarter and more accurate. In a recent retail for example we were able to improve an AI model used for predicting sales trends by training it more effectively by incorporating the active metadata. Improvement to the metadata mean’t details like seasonality, promotional events, and regional preferences, ensured that the AI could understand these patterns better.
Improving Data Fabric - think of data fabric like a smart network that connects all your data together by understanding all the joins and connections, making it easier to manage and use. Active metadata acts as the real-time map and guide for this network, showing how data is used and performing, ensuring everything runs smoothly and efficiently. In a healthcare setting data fabric supported by active metadata means you can leverage the integrated patient data from various sources like electronic health records, lab results, and wearable devices. The active metadata part provides real-time insights into data usage and performance such as tracking how often certain patient data is accessed by doctors or flagging any inconsistencies or data entry errors, ensuring a much more proactive and accurate approach to patient care.
Enabling Better Decision Making - Active metadata also provides deep insights into your data, much like a detailed report on how things are going in your business. Considering that most data savvy organisations now consider data a strategic asset, having insights into its overall health and performance suddenly becomes quite an imperative. This data helps business leaders make informed decisions, using AI to its fullest potential for better outcomes. In finance client this was being used to identify trends such as peak transaction times, common transaction types, and potential fraud patterns. This information helped the bank make better decisions about resource allocation, customer service improvements, and fraud prevention measures.
Best Practices for Metadata Management
To effectively manage metadata and leverage it for AI, business should consider the following best practices:
Establish Clear Metadata Standards: Implement standardised metadata practices across the business to ensure consistency and interoperability.
Invest in Advanced Metadata Management Tools: Make use of the advanced tools and technologies that support active metadata management and integration with AI systems.
Foster a Culture of Data Governance: Encourage a culture of data governance where all stakeholders understand the importance of metadata and actively contribute to its management.
Regularly Update and Maintain Metadata: Ensure that metadata is continuously updated and maintained to reflect changes in data and business processes.
Leverage AI for Metadata Management: Use AI and machine learning to automate metadata management tasks, such as metadata extraction, tagging, and classification.
Data Strategy and Metadata Management (MDM)
As I alluded to at the start of this post, Metadata Management (MDM) is often overlooked or dismissed as a technical detail rather than a strategic necessity.
However, neglecting MDM can lead to significant challenges that undermine your data strategy's effectiveness.
As such it’s really important that you work on these challenges from the outset and your Data Strategy is as good a place to start as any.
With MDM being frequently viewed as a highly technical and complex process that is too cumbersome for business leaders to worry about means that it can be unintentionally excluded from broader strategic discussions, leaving it as a low-profile task relegated to IT departments.
However, this approach is terribly shortsighted and will impede your ability to harness the full power of its data.
By explicitly including MDM in your data strategy, you raise its profile within the business.
This inclusion sends a clear message that managing master data is not just an IT concern but a fundamental business priority.
It fosters awareness and understanding among senior management and business units about the importance of clean, consistent data.
Last Word
If I was to offer one hint on top of the above it would be to select the individuals responsible for MDM carefully.
Without wishing others be unkind, but all too often we see MDM being delegated to someone in IT who isn’t necessarily wired the right way to lead the charge and be the catalyst for change.
Successful MDM requires educating from the top of the business down and needs someone with the personality and communication skills to sell the dream and the drive to chivvy everyone along and get on the bus.